Well, let’s hope this post is not then. Recently, my brother texted a quote from a meteorology text book: “If I had only one more day left to live, I would live it in my statistics class – it would seem so much longer”**. Ooch, this hurts. Why do non-statisticians find the fascinating field of statistics so boring? The answer is simple, and I think we all know it: because the way statisticians teach statistics to others – it is often very, very boring. Here’s my, likely incomplete, list of causes.
Why is it boring?
1. We teach recipes. Or call them rules-of-thumb, guidelines, whatever. Statistics is marketed as a decision tool: p <= 0.05, bingo. But you know what: the best cooks don’t follow the recipe. They think for themselves. Moreover, once you have seen the construction of, say, one test statistic, it is boring to discuss seventeen other ones for slightly different settings.
2. We teach what is easy to test, not what is actually relevant. Often our questions are on calculations for which students have to use formulas they don’t really understand. And math is definitely very boring when you don’t understand what you’re doing. Why don’t we focus more on concepts for the non-math inclined students? I really feel for many students it is way more important to spend three lectures on what a p-value really is than to spend ten minutes on its definition and two-and-half hour on a large variety of test statistics.
3. We teach uncertainty with too much certainty. We tell students about the concepts, often as these are taken for granted by the entire community. But we should tell them about our quarrels, our fierce debates, our fights even. We don’t always agree, and let’s share that. Tell them about the p-value debates, tell them about Bayesian vs frequentist views points, tell them even about Fisher vs Neyman for the historical perspective. Yes, it’s confusing, but it’s fun too.
4. We don’t brag enough about our own discipline. The impact of our discipline on science has been tremendous. Show those examples. Start with Florence Nightingale. She knew how to sell her statistical ideas very well. Students are definitely more interested in technical matters if they know what these matters have brought about.
5. We shy away from discussing the fantastic and often mind-boggling phenomena that we observe and understand better due to statistics. Think of regression to the mean, selection bias, overfitting, type-I error inflation, Lindley’s paradox. It strikes me when we explain one of our major topics, regression analysis, we often don’t talk about its origin, the regression to the mean phenomenon.
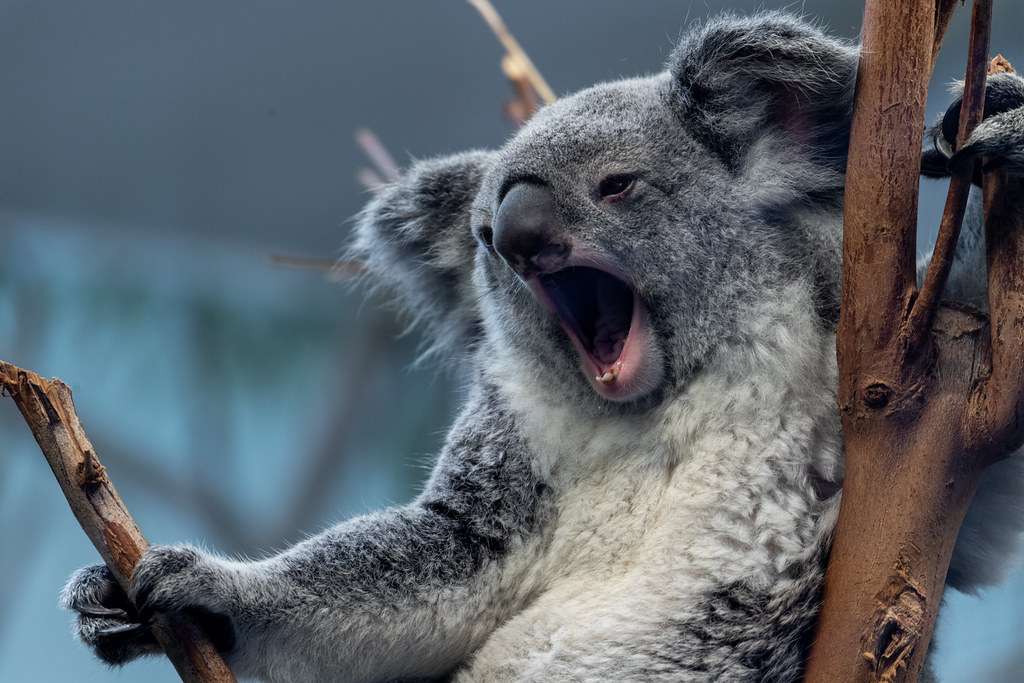
Dazzling accuracies
An example of a story that I tell medicine students to motivate them. A bit condensed here. In the late 90s, the genomics revolution allowed researchers to simultaneously measure a vast number of genes, rendering so-called high-dimensional data: data with more variables than samples. The first results of using these data for diagnostic purposes were amazing: all of a sudden one could classify who would or would not benefit from treatment with dazzling accuracies like 97%. Results were published in top journals, and for a short period we believed these ‘biomarkers’ would make a giant impact on medicine.
But then people started to validate the results: accuracies dropped rigorously to disappointing figures like 65%. Meanwhile statisticians figured out what the problem was. In those days, researchers often used so-called gene filtering methods. Simply put: they used ALL samples to pre-test which genes correlate to the outcome. And only these genes were used when training the classifier. If one studies a few genes only – as we were used to do – this strategy is fine. But here comes the statistical insight: if you do this in high dimensions, you’re bound to strongly overfit, even if you do split samples when building the classifier.
Statisticians to the rescue
Fortunately, several statisticians took the effort to write about the issue in high-profile journals, which helped to eradicate this bad research practice. And others developed clever solutions that incorporated the gene selection into the classifier, such as lasso, elastic net and variations thereof, nowadays part of main stream science. It seems to me that this is an example of the huge scientific footprint that statistics has had.
When I tell medicine students this story, they may not understand exactly what’s going on, but they do get the main idea: a fishing expedition in a big pond with many rods is likely to render too optimistic results. Here’s a reaction by one of them during the coffee break: “I really did not expect that statistics is actually so important”. Still makes my day!
Less is more
I’m a bit of a storyteller – there’s a reason why I write blog posts – and that may not be everyone’s cup of tea. But hopefully the general rule ‘less is more’ can help all of us to make statistics classes a lot more interesting and educational in the long run. Fewer recipes, fewer tricks, traded for more context, more juice, more enthusiasm. Shouldn’t be too hard.
*I’m 90% confident that this statement holds true for at least 95% of the population
**Anonymous [from C.C. Gaither (Ed), 1996: Statistically Speaking: A Dictionary of Quotations, Inst of Physics Pub, 420 pp]
Foto’s: Flickr
Add comment